There’s been a lot of talk lately about new technologies used to advance critical areas of medicine. Namely, with infectious diseases, and specifically the Covid-19 crisis, people are discussing the potential of artificial intelligence to speed up vaccine creation and drug discovery.
In recent weeks, unrelated to the novel coronavirus, we have seen major news from MIT, that a brand new type of antibiotic, halicin, has been created through use of a program based on machine learning. This new drug is capable of eradicating bacteria, like antibiotic-resistant E.coli, that even some of today’s most powerful antibiotic medicines such as ciproflaxicin are unable to kill. Scientists claim that this new drug, pending clinical trials, has the promise to be among the most powerful of its type of drug ever discovered. Furthermore, there is evidence that even after thirty days of exposure, bacteria are unable to develop a resistance to this new drug. Of course, identifying a candidate drug is only the first step in a long, expensive process of clinical trials to establish safety and efficacy in humans, but if its early promise holds true, halicin could offer a major advance for infectious disease medicine. Machine learning helps this process of drug discovery go faster by making the job of sorting through countless drug candidates, and identifying the ones with the greatest potential, much easier than the relatively manual way it has been done in the past.
Recently, there have been a number of headlines claiming that a Covid-19 vaccine may be available and mass produced within the next year to year and a half. Developing a vaccine has traditionally meant utilizing various parts of dead/weak viruses, and purifying them to inject into healthy people so that their immune systems will be able to recognize the pathogens and develop an immunity against them. This method that has been used for decades. More recent approaches seek to identify smaller pieces of a virus that can lead to immunity without creating the risk of infection. For example, “subunit” vaccines use peptides; nucleic acid vaccines (like one candidate vaccine against the virus that causes covid-19 currently beingtested in the US) incorporate segments of viral DNA or RNA. Given how complex it can be to analyze biomolecules, “subunit” and nucleic acid based approaches have the potential to benefit significantly from the computational advantages of artificial intelligence.
In addition, there is much debate about the use of AI in diagnosis and prognosis of Covid-19 using lung scans. Because the severe respiratory infections caused by coronavirus often show tell-tale microscopic shapes within human lungs, it may be possible to use visualization such as X-rays, CAT scans and MRIs to make a diagnosis and estimate a patient’s prognosis. This is not straightforward, as other kinds of pneumonia and even congestive heart failure can present similar images, and X-rays often look much worse than the patient does on presentation. Basically, a diagnosing doctor could use a lot more experience and expertise in examining these images than one radiology department in one hospital can provide. AI and machine learning can potentially collate images and information about the clinical course of thousands of cases seen worldwide in the last couple of months. Such comparison and analysis could help predict which patients need hospitalization and certain types of treatment such as ventilators. What we don’t know yet is whether such testing and analysis will be more cost-effective than simply examining patients and checking how well they are getting oxygen into their blood with a simple pulse oximeter. Just last year, the FDA approved the US’ first AI-based X-ray system, developed by GE to help detect pneumothorax, in as little as 15 min – a big reduction from the previous norm which was 8 hours or more (and sometimes, not nearly as accurate).
Why is AI able to make advances in medicine that scientists have not been able to do on their own all these years?
A lot of it has to do with speed. Being able to do math faster and more like a human allows virtual experiments and modeling to be done on a large scale much quicker than ever before. With today’s pharmaceutical experiments and clinical trials costing so much, it is often preferable to do “in-silico” experiments before “in vivo” ones (which is a fancy way of saying that it’s much cheaper to make a artificial medical models/simulations on a computer – “in silico”– to rule out drug candidates with problems before committing to expensive lab equipment and animal/human trials, “in-vivo”). In recent years, computers have been able to gain substantial achievements in artificial intelligence, thanks in large part to increases in CPU and GPU (central processing unit and graphics processing unit) speed advances. Furthermore, large user communities across the globe have worked together to contribute to the development of robust, open source AI platforms such as Tensorflow , Pytorch and Keras, to make new, fast AI algorithms and sample programs available to research communities, making development of new medical machine learning programs faster than ever before.
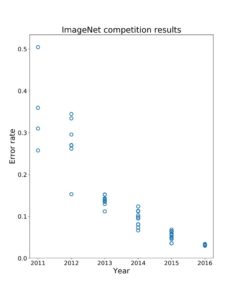
As an example of AI’s recent general improvements, one of the most popular image classification AI databases, known as “Imagenet,” is used in annual competitions to see how good new AI systems are at sorting its images. The best systems classified images wrongly about ~26% of the time in 2011 (at best), but by 2016, was able to achieve error rates of only about 3.1%. (By contrast, humans themselves show an average error rate of about 5% in identifying the same objects). In the past few years, this error rate has fallen even further, so that now it hovers around 1%. This remarkable improvement in AI happened in such a short period of time, and based on studies in different areas of medical AI technology, it is thought that AI has the potential to be better than human doctors at making various kinds of visual medical diagnoses (as can be seen here in this AAMC News article: https://www.aamc.org/news-insights/will-artificial-intelligence-replace-doctors) For instance, IBM’s AI-based Watson system is able to diagnose heart disease earlier than many cardiologists, up to two years earlier in fact, in studies funded by NIH and performed by Sutter Health and Geisinger Health System in association with IBM (https://mashable.com/2017/02/22/ibm-watson-clinical-imaging-cardiology/ and https://www.ibm.com/blogs/research/2017/04/using-ai-to-predict-heart-failure/)
It is an exciting time in AI, as the scientific community makes significant advances in a number of core algorithms and models.. Very quickly, these advances are shared in the research community and make their way very quickly in different STEM fields, such as medicine. In the few months since it emerged Covid-19 has already become a major target of global AI-based medical research, and promising progress is already being made. It is only a matter of time before AI outpaces doctors and scientists in not only the diagnosis of diseases, but also the discovery of new treatments–maybe even an effective medication for Covid-19 in the near future.
Check out Tumblehome’s K-12 COVID educational resources page here, with totally free infographics, video games, card game templates, videos and more: https://www.tumblehomebooks.org/coronadownloads